
PUBLICATIONS
Published works
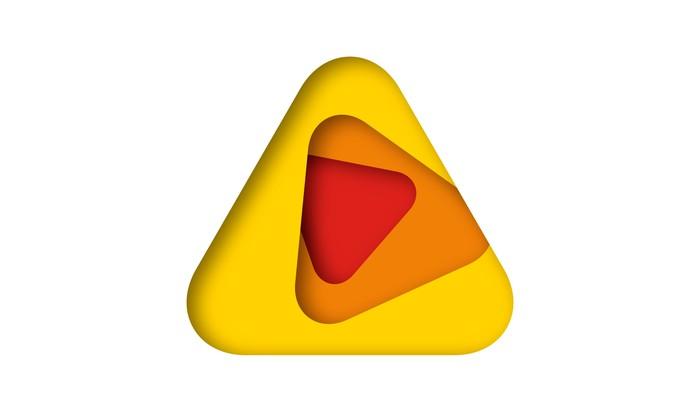
Improving flood forecast skill using remote sensing data
Title | Improving flood forecast skill using remote sensing data |
Publication Type | Conference Paper |
Year of Publication | 2017 |
Authors | Li, Y, Grimaldi, S, Wright, A, Walker, J, Pauwels, V |
Conference Name | AFAC17 |
Date Published | 09/2017 |
Publisher | Bushfire and Natural Hazards CRC |
Conference Location | Sydney |
Abstract | Floods are among the most important natural disasters in Australia. The average annual cost of floods in the last 40 years has been estimated to amount to $377 million, with the 2010-2011 Brisbane and South-East Queensland floods alone leading to $2.38 billion in economic damage, and 35 confirmed deaths. Flood forecasting systems are the most important tools to limit this damage, but are prone to a considerable degree of uncertainty. During the last decades, significant research focusing on the monitoring of the global water cycle through satellite remote sensing has been performed. The strength of remote sensing is the opportunity to provide information at large spatial scales, including areas that are difficult or impossible to monitor using on-ground techniques. For these reasons it is believed that the use of remote sensing data can improve the quality of operational flood forecasts. Operational flood forecasting systems typically consist of a hydrologic model, which estimates the amount of water entering a river system, and a hydraulic model, which models the flow of water inside the river system. However, hydrological and hydraulic models are prone to a significant degree of uncertainty and error, caused by errors and uncertainties in the initial conditions, meteorological forcing data, topographic data, and model errors and/or oversimplification (Li et al., 2016; Grimaldi et al., 2016). In order to reduce this predictive uncertainty, we propose to constrain the models using remote sensing data. In particular, remotely sensed soil moisture data are being used to improve the hydrologic model results, while remotely sensed water levels and/or flood extent data can be used to support the hydraulic model implementation, calibration and real time constraint. The project focusses on two test sites, the Clarence River in New South Wales and the Condamine-Balonne River in Queensland. Figure 1 shows an overview of these test sites. Initial catchment soil moisture plays an important role in controlling runoff generation and infiltration processes, which consequently impact streamflow forecasting. Recent development in remote sensing techniques provide a new potential to monitor spatially distributed surface soil moisture. As a result, soil moisture assimilation for flood forecasting has been a hot research topic in the resent years. The ensemble Kalman filter (EnKF) has been widely used for soil moisture assimilation by the scientific and operational communities, due to its relatively satisfactory efficacy and efficiency. However, one of the challenge is that streamflow forecasts are calculated not only from current states, but also from antecedent states in many hydrologic models, while the EnKF updates the current states only, and so may not achieve an optimal performance. As an alternative, assimilation of surface soil moisture by the ensemble Kalman smoother (EnKS) has been demonstrated to give better soil moisture reanalysis (Dunne et al., 2006). Nevertheless, the impact of the EnKS-based soil moisture assimilation on flood forecasting remains a research question. |
Refereed Designation | Non-Refereed |

Published Works
